UNE Specifications – Data Quality Assessment Guide
Source: Gobierno de España
Today, data quality plays a fundamental role in the modern world, where information is a valuable asset. Ensuring that data is accurate, complete, and reliable has become essential for the success of organizations and ensures the success of informed decision-making.
The quality of data has a direct impact not only on the internal exchange and use within each organization but also on the sharing of data between different entities, being a key variable in the success of the new paradigm of data spaces. When data is of high quality, an environment is created for the exchange of accurate and consistent information, enabling organizations to collaborate more effectively, fostering innovation, and jointly developing solutions.
Good data quality facilitates the reuse of information in different contexts, generating value beyond the system that creates them. High-quality data is more reliable and accessible, and can be used by multiple systems and applications, increasing its value and utility. By significantly reducing the need for constant corrections and adjustments, time and resources are saved, allowing for greater efficiency in project implementation and the creation of new products and services.
Data quality also plays a fundamental role in advancing artificial intelligence and machine learning. AI models rely on large volumes of data to obtain accurate and reliable results. If the data used is contaminated or of low quality, the results of AI algorithms will be unreliable or even erroneous. Therefore, ensuring data quality is essential to achieve the maximum performance of AI applications, reduce or eliminate biases, and leverage their full potential.
With the aim of offering a process based on international standards that can help organizations use a quality model and define appropriate quality characteristics and metrics, the Data Office has sponsored, promoted, and participated in the creation of Specification UNE 0081 Data Quality Assessment, complementing the existing Specification UNE 0079 Data Quality Management, which is more focused on defining data quality management processes than on data quality itself.
UNE Specifications – Data Quality Assessment Guide
Specification UNE 0081, part of the ISO/IEC 25000 family of international standards, allows for understanding and evaluating the quality of data within any organization. It enables the establishment of a future plan for improvement and even the formal certification of data quality. The intended audience for this specification, applicable to any type of organization regardless of its size or focus, includes data quality managers, as well as consultants and auditors who need to conduct assessments of datasets within their roles.
The specification first presents the data quality model, detailing the quality characteristics that data can have, along with some applicable metrics. Once this framework is defined, the specification then outlines the process to be followed to assess the quality of a dataset. Finally, the specification concludes by detailing how to interpret the results obtained from the assessment, providing concrete examples of application.
Data Quality Model
The guide proposes a series of quality characteristics following those present in the ISO/IEC 25012 standard, classifying them into those inherent to the data, those dependent on the system where the data is hosted, or those dependent on both circumstances. The choice of these characteristics is justified as they encompass those found in other reference frameworks such as DAMA, FAIR, EHDS, IA Act, and GDPR.
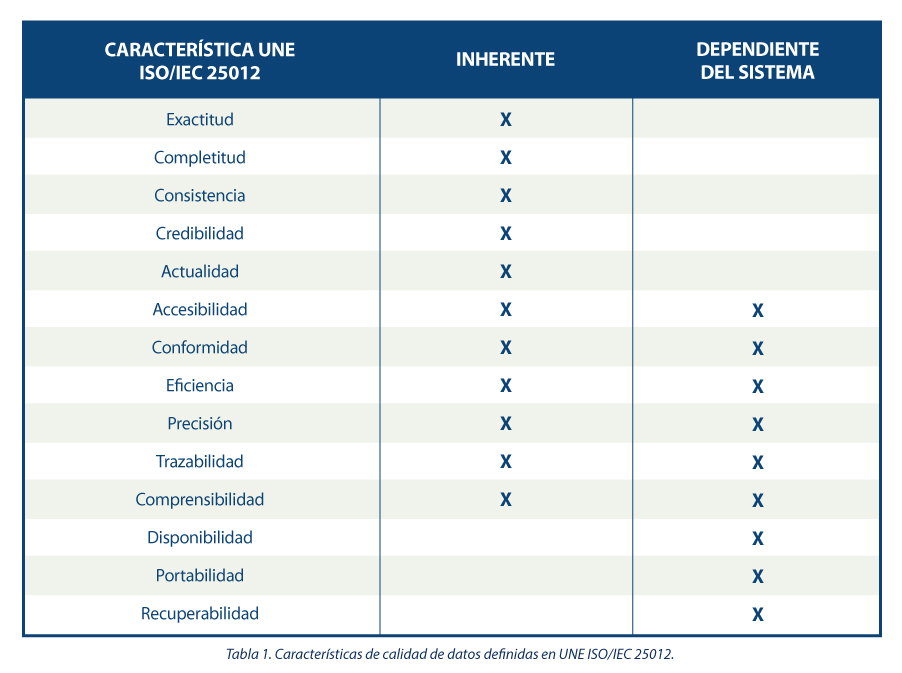
Based on the defined characteristics, the guide relies on the ISO/IEC 25024 standard to propose a set of metrics that serve to measure the properties of these characteristics, understanding these properties as “subcharacteristics” of the characteristics.
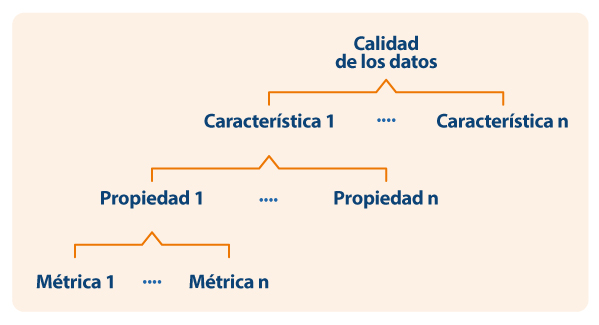
Thus, by way of example, following the dependency scheme, for the specific characteristic of “consistency of data format,” its properties and metrics are presented, with one of them being detailed.
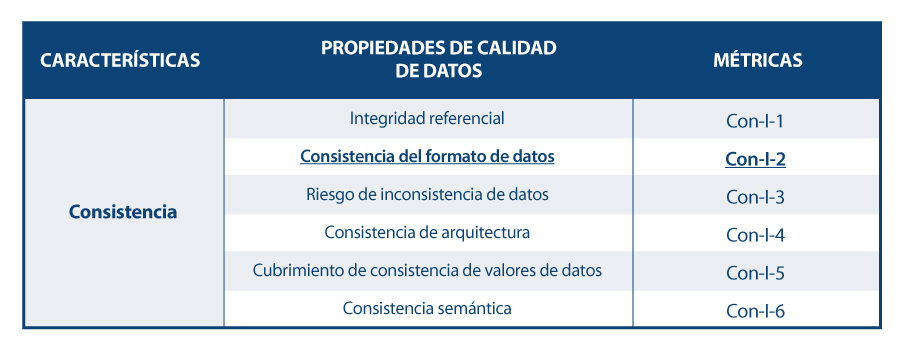
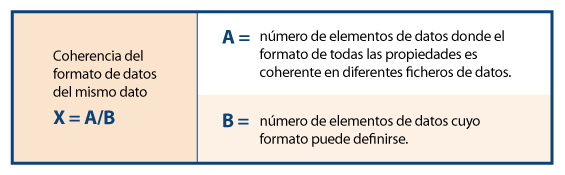
Process for Assessing the Quality of a Dataset
To carry out the actual assessment of data quality, the guide proposes following the ISO/IEC 25040 standard, which establishes an evaluation model that takes into account both the requirements and constraints defined by the organization, as well as the necessary material and human resources. With these requirements, an evaluation plan is established through specific metrics and decision criteria based on business requirements, enabling the accurate measurement of properties and characteristics and the interpretation of their results.
Below is an outline of the steps in the process, along with its main activities:
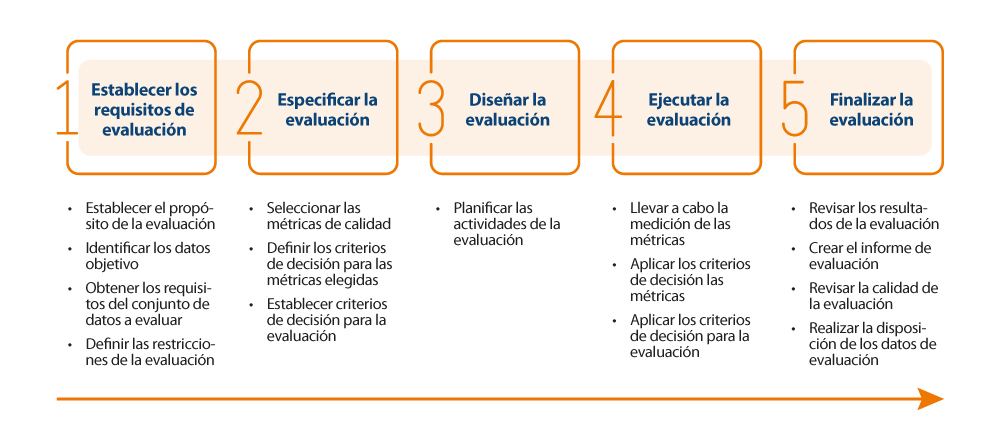
Quality Assessment Results
The result of the assessment will depend directly on the requirements set by the organization and the compliance criteria. The properties of the characteristics are usually evaluated on a scale of 0 to 100 based on the values obtained in the metrics defined for each of them. The characteristics, in turn, are evaluated by aggregating the former, also on a scale of 0 to 100, or by converting to a discrete value of 1 to 5 (1 being poor quality, 5 being excellent quality) based on the calculation and weighting rules that have been established. Just as the measurement of properties yields that of characteristics, the same applies to these characteristics. Through their weighted sum based on the defined rules (assigning more weight to some characteristics than others), a final result of data quality can be obtained. For example, if we want to calculate data quality based on a weighted sum of its intrinsic characteristics, where, due to the nature of the business, it is important to give more weight to accuracy, then a formula could be defined as follows:
Data Quality = 0.4Accuracy + 0.15Completeness + 0.15Consistency + 0.15Credibility + 0.15*Timeliness
Suppose that in a similar manner, each of the quality characteristics has been calculated based on the weighted sum of its properties, resulting in the following values: Accuracy=50%, Completeness=45%, Consistency=35%, Credibility=100%, and Timeliness=50%. In this way, data quality would be:
Data Quality = 0.450% + 0.1545% + 0.1535% + 0.15100% + 0.15*50% = 54.5%
If the organization has established requirements as shown in the following table:
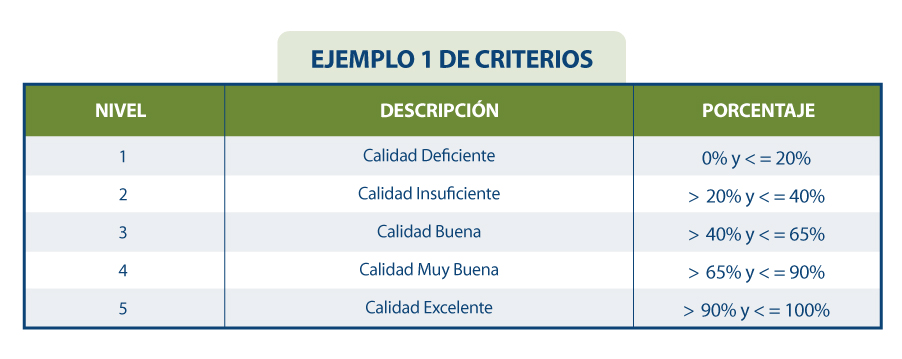
It could be concluded that the organization, overall, has a data rating of “3 = Good Quality.”
In summary, the evaluation and improvement of dataset quality can be as thorough and rigorous as necessary and should be carried out iteratively and consistently. This ensures that data continually increases in quality, guaranteeing a minimum data quality or even the possibility of certification. This minimum data quality can involve enhancing datasets internal to an organization—those the organization manages and exploits for the operation of its business processes. Alternatively, it can be used to facilitate the sharing of datasets through the new paradigm of data spaces, creating new market opportunities.
In the latter case, when an organization intends to integrate its data into a data space for future intermediation, it is advisable to conduct a quality assessment, appropriately labeling the dataset in reference to its quality, perhaps through metadata. Data with proven quality has a distinct utility and value compared to data lacking it, positioning the former favorably within the competitive market.